Site menu:
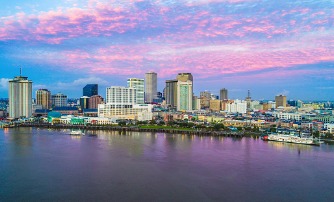
OPT2022
We welcome you to participate in the 14th International OPT Workshop on Optimization for Machine Learning, to be held as a part of the NeurIPS 2022 conference. This year we particularly encourage (but not limit) submissions in the area of Reliable Optimization Methods for ML.
We are looking forward to an exciting in-person OPT!
The Workshop
Optimization lies at the heart of many machine learning algorithms and enjoys great interest in our community. Indeed, this intimate relation of optimization with ML is the key motivation for the OPT series of workshops. We aim to foster discussion, discovery, and dissemination of state-of-the-art research in optimization relevant to ML.
To foster the spirit of innovation and collaboration, OPT 2022 will focus the contributed talks on topics in the area of Reliable Optimization Methods for ML:
Many optimization algorithms for ML were originally developed with the goal of handling computational constraints (e.g., stochastic gradient based algorithms). Moreover, the analyses of these algorithms followed the classical optimization approach where one measures the performances of algorithms based on (i) the computation cost and (ii) convergence for any input into the algorithm. As engineering capabilities increase and the wide adoption of ML into many real world usages, practitioners of ML are seeking optimization algorithms that go beyond finding the minimizer with the fastest algorithm. They want reliable methods that solve real-world complications that arise.
For example, increasingly bad actors are attempting to fool models with deceptive data. This leads to questions such as what algorithms are more robust to adversarial attacks and can one design new algorithms that can thwart these attacks? The latter question motivates a new area of optimization focusing on game-theoretic environments, that is, environments where there are competing forces at play and devising guarantees. Beyond this, a main reason for the success of ML is that optimization algorithms seemingly generate points that learn from training data; that is, we want minimizers of training data to provide meaningful interpretations on new data (generalization) yet we do not understand what features (e.g., loss function, algorithm, depth of the architectures (deep learning), and/or training samples) yield better generalization properties. These new areas of solving practical ML problems and their deep ties to the optimization community warrants a necessary discussion between the two communities.
Specifically, we aim to discuss the meanings of generalization as well as the challenges facing real-world applications of ML and the new paradigms for optimizers seeking to solve them. By bringing together optimizers with experts in statistics and ML applications, OPT 2022 will foster insightful discussions around these topics and more.
The OPT2022 Organizers (preferred contact email: optmlworkshop@googlegroups.com)
- John Duchi (Stanford)
- Quanquan Gu (UCLA)
- Cristóbal Guzmán (Pontificia Universidad Católica de Chile)
- Courtney Paquette (head) (McGill University)
- Sebastian Stich (CISPA Helmholtz Center for Information Security)
Registration
For registration and pricing see NeurIPS.cc.
Important Dates
- Deadline for submission of papers: September 30, 2022 (AoE)*
- Notification of acceptance: October 20, 2022
- Presentation upload to slideslive: Nov 10, 2022)
- Camera-ready papers upload to openreview: Nov 10, 2022 (OPT2022 style file required)
- Workshop date: December 3, 2022